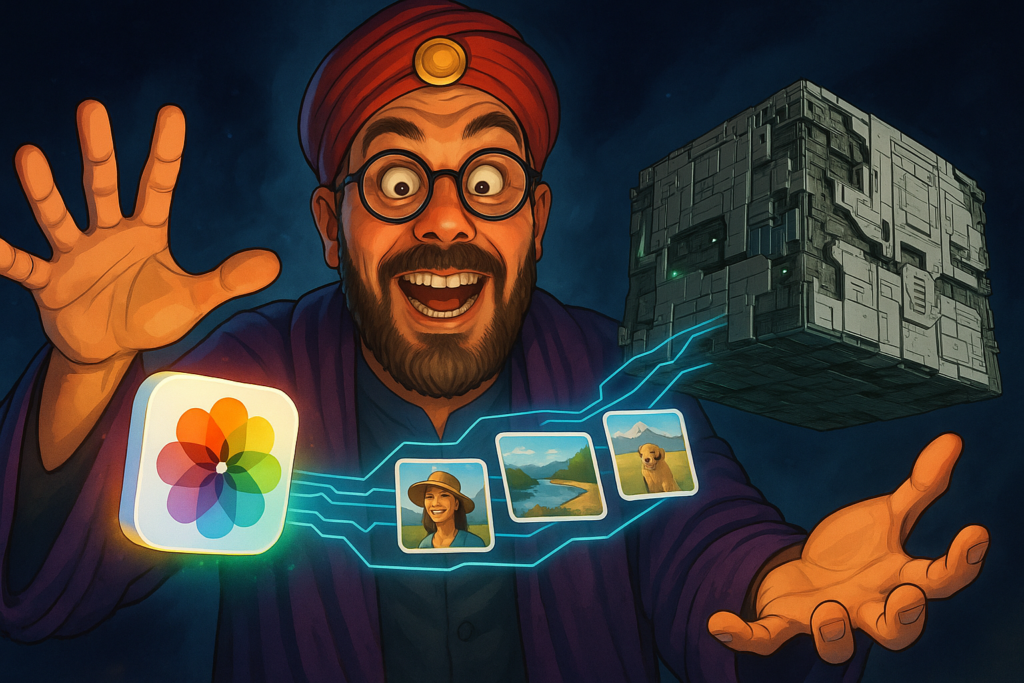
In my last post I gave an introduction to my overall hardware and software setup for backup. But this was the easy part. Backing up several massive Apple Photos libraries—each overflowing with iPhone snapshots, RAW captures from my system camera, videos, panoramas, and AI-generated art—turns out to be a far more complex endeavor than initially anticipated.
For the impatient… You can find the resulting repository, with one iOS solution (which does not completely satisfy my requirements) as well as a macOS solution (which only runs if my MacBook is “awake”, but besides that is an A+), here: https://github.com/juangamnik/apple_photos_backup/
Still with me? Great, let’s dive into the story… Over months of testing, scripting, and wrestling with encrypted storage quirks, a reliable, fully automated solution has finally taken shape. In this post, I’ll walk you through the entire journey: from the early missteps to the final macOS-based workflow that ensures every original file (complete with sidecar and edit metadata) eventually lands safely in an encrypted, deduplicated long-term archive. Whether you’re juggling multiple family libraries or simply want bulletproof Apple Photos backups, I hope this detailed account of trials and triumphs helps you avoid the pitfalls and understand exactly how the tooling works.
Disclaimer: This issue vanishes, if you have a windows or macOS device running 24/7 with enough disk space to deactivate “optimize space”. If true: don’t read 🤓.
By the way: Apple encourages you to backup your iCloud Photos!